
\[f(x) = \frac\), and the Variance is a bit more complex it’s usually written in terms of the Expectation.
#Gamma cdf pdf#
If \(X \sim Beta(a,b)\), the PDF is given by: Remember that, like the Uniform, the Beta has two parameters \(a\) and \(b\) (often called \(\alpha\) and \(\beta\)). Now that we have sort of an idea of what the Beta looks like (or, more importantly, has the potential of looking like, since we know that it can change shape) let’s look at the PDF. Remember, though, that no matter the shape, the Beta i continuous and is always bounded between 0 and 1 (the support is all values from 0 to 1). You can manipulate the shape of the distribution of the Beta just by changing the parameters, and this is what makes it so valuable. If \(a=b=2\), you get a smooth curve (when you generate and plot random values). For example, if you allow \(a=b=1\), then you get a Standard Uniform distribution. Changing the parameters \((a,b)\) (which you might also see as \(\alpha\) or \(\beta\), and are the two parameters that the Beta takes, like the Normal takes \(\mu\) and \(\sigma^2\)) actually changes the shape of the distribution drastically. In fact, in general, the Beta does not take on any one specific shape (recall that the Normal is bell-shaped, the Exponential right-skewed, etc.). That’s where the ‘generalization’ comes in: it shares these key properties (continuous and bounded) but is generalized to different shapes (not just flat like the Uniform). However, it is (usually) not flat or constant like the Uniform. The Beta, in turn, is also continuous and always bounded on the same interval: 0 to 1. The Uniform, as we know, has a bounded interval, often between 0 and 1 (the Standard Uniform). Think about this for a moment the rest of the continuous random variables that we have worked with are unbounded on one end of their supports (i.e., a Normal can take on any real value, and an Exponential can go up to infinity). The Uniform is interesting because it is a continuous random variable that is also bounded on a set interval. What exactly is a generalization of a distribution? Well, let’s first consider the Uniform distribution itself. Instead, probably the most common story for the Beta is that it is a generalization of the Uniform.

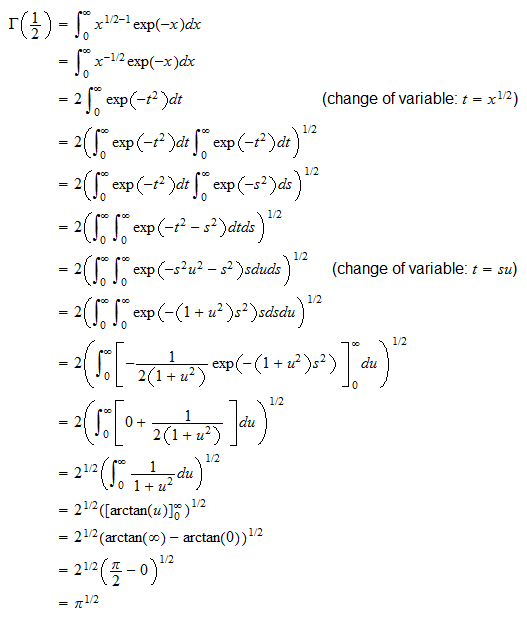
Again, you could think of the Poisson as the number of winning lottery tickets, or the Hypergeometric as drawing balls from a jar, but there’s really not any extremely relevant real-world connections for the Beta. The issue with the Beta that likely contributes to its ‘aura of difficulty’ is that it doesn’t necessarily have a ‘cute,’ simple story that helps to define it. It helps to think of the Binomial as a set number of coin flips, or the Exponential as the time waiting for a bus. We’ve often tried to define distributions in terms of their stories by discussing what they represent in practical terms (i.e., trying to intuit the specific ‘mapping’ to the real line), we get a better grasp of what we’re actually working with. 9 Limit Theorems and Conditional Expectation.Expectation, Indicators and Memorylessness.
